The Ultimate Guide to Data Reliability:
Everything You Need to Know
Kylo B
10/15/2024
The Ultimate Guide to Data Reliability: Everything You Need to Know
In today's data-driven world, data reliability is paramount for making informed business decisions, maintaining operational efficiency, and building trust. Organizations across industries rely on vast amounts of data to inform strategies, automate processes, and fuel AI models. However, data that is unreliable—incorrect, outdated, or inconsistent—can lead to costly mistakes, missed opportunities, and compromised trust. This comprehensive guide delves into the fundamentals of data reliability, the common challenges organizations face, and best practices for ensuring your data is dependable.
What is Data Reliability?
Data reliability refers to the ability of data to consistently represent accurate, complete, and trustworthy information over time. Reliable data is crucial for businesses to make sound decisions, develop analytics models, and comply with regulatory standards. In short, reliable data ensures that the information organizations depend on remains usable, consistent, and aligned with reality.
Key Components of Data Reliability
To truly understand what makes data reliable, it’s essential to break down the key components:
Accuracy: Data must correctly represent the real-world event or object it is supposed to describe. Inaccurate data can lead to incorrect conclusions and flawed decisions.
Consistency: Data should be consistent across various systems, platforms, and databases. For example, if a customer’s name or transaction details appear differently in two systems, this lack of consistency can lead to errors.
Completeness: Reliable data must be complete, with no missing information. Incomplete data can skew analysis and lead to erroneous insights.
Timeliness: Data should be up-to-date and available when needed. Stale or outdated data can be as harmful as incorrect data, especially in fast-paced industries where real-time information is critical.
Integrity: This involves ensuring that data is maintained in a way that preserves its accuracy and consistency over its lifecycle. Data integrity is crucial for maintaining trust in the data.
Common Challenges to Data Reliability
Despite its importance, achieving data reliability can be difficult. Organizations often face the following challenges:
1. Data Silos
Data silos occur when information is stored in separate systems or departments that do not communicate with each other. This fragmentation can lead to inconsistencies in data and create reliability issues when trying to consolidate and analyze it.
2. Human Error
Manual data entry or improper data handling can introduce errors that affect data reliability. Even small mistakes, such as a typo or incorrect formatting, can have significant downstream effects.
3. Outdated Data
As time passes, some data can become obsolete. Outdated data can lead to inaccurate decision-making, particularly in industries where real-time information is critical, like finance or e-commerce.
4. Data Corruption
Technical issues, system failures, or software bugs can corrupt data, making it unusable or unreliable. Without proper backups and redundancy measures, corrupted data can result in permanent loss.
5. Lack of Standardization
Different systems may store the same data in various formats or under different naming conventions. This lack of standardization creates challenges when trying to merge, analyze, or transfer data across platforms.
Best Practices for Ensuring Data Reliability
Ensuring the reliability of your data requires a proactive approach that involves policies, procedures, and technologies designed to prevent errors and maintain consistency. Here are key best practices to follow:
1. Establish a Data Governance Framework
A well-defined data governance framework ensures that data is managed and used consistently across the organization. This includes establishing rules for data entry, formatting, storage, and access. Clear ownership and accountability for data quality should be defined at all levels of the organization.
2. Implement Automated Data Validation and Cleaning
Automating the processes of data validation and data cleaning can significantly reduce human errors and improve reliability. Tools that automatically detect and correct discrepancies, duplicates, or missing information ensure that data is consistently accurate and complete.
3. Use Data Redundancy and Backup Systems
Redundancy ensures that multiple copies of data exist across different storage systems or locations. In the event of system failure or data corruption, these backups can be used to restore lost or damaged data. Regular automated backups, combined with redundancy, protect against both technical failures and accidental data loss.
4. Establish Clear Data Standardization Protocols
Standardizing data collection, entry, and storage protocols ensures consistency across different departments and systems. Common standards for naming conventions, file formats, and data structures reduce the risk of data discrepancies.
5. Monitor and Audit Data Regularly
Data auditing involves regular reviews of data to check for consistency, accuracy, and completeness. Automated monitoring tools can track data usage and flag potential issues in real time, allowing you to resolve problems before they affect business operations.
6. Invest in High-Quality Data Management Tools
There are numerous tools available to help businesses maintain data reliability. Data management platforms (DMPs), Master Data Management (MDM) systems, and Enterprise Resource Planning (ERP) software offer robust features for managing and ensuring the integrity of data across an organization.
7. Train Employees in Data Best Practices
Since human error is one of the leading causes of data reliability issues, it’s important to ensure that employees are properly trained in data management best practices. This includes understanding how to correctly input, handle, and store data, as well as how to identify and report potential data issues.
Industry Standards for Data Reliability
Adhering to industry standards and frameworks can further ensure data reliability across the organization:
ISO/IEC 25012: Data Quality Model: This standard outlines key data quality characteristics, including reliability, accuracy, and completeness, offering a framework for assessing and improving data reliability.
GDPR (General Data Protection Regulation): For organizations that process personal data, adhering to GDPR guidelines helps ensure data is collected, stored, and processed in a way that maintains its integrity and reliability.
HIPAA (Health Insurance Portability and Accountability Act): In the healthcare sector, HIPAA mandates strict guidelines for handling data to ensure it remains secure, accurate, and accessible.
SOX (Sarbanes-Oxley Act): In the financial industry, SOX sets requirements for data reliability to ensure accurate reporting and auditing, particularly for publicly traded companies.
The Role of Technology in Data Reliability
The rise of new technologies has greatly impacted how organizations can achieve and maintain data reliability:
1. Artificial Intelligence (AI) and Machine Learning (ML)
AI and ML algorithms are increasingly used to identify data anomalies, clean data, and improve data consistency. These technologies can also predict and prevent potential data errors by analyzing large datasets in real time.
2. Blockchain for Data Integrity
Blockchain technology offers a decentralized way to store and verify data, ensuring it cannot be altered or tampered with. This guarantees that data remains reliable over time, particularly in industries like finance and supply chain management.
3. Cloud Storage Solutions
Cloud-based storage solutions provide redundancy and scalability, making it easier for organizations to maintain reliable data across multiple locations. Many cloud platforms also offer built-in data validation and backup services to further enhance reliability.
Ensuring data reliability is not just a technical necessity—it is a strategic advantage. Reliable data fuels better decision-making, builds trust with stakeholders, and reduces the risk of costly errors. By understanding the key components of data reliability, addressing common challenges, and implementing best practices and technologies, organizations can maintain data that is consistent, accurate, and dependable over time.
To stay competitive in today’s data-centric world, businesses must prioritize data reliability at every stage, from data collection to storage, analysis, and reporting. The future of data-driven organizations hinges on the reliability of the information they depend on to make decisions, drive growth, and maintain customer.
Subscribe to our newsletter
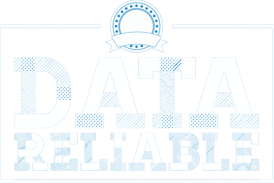
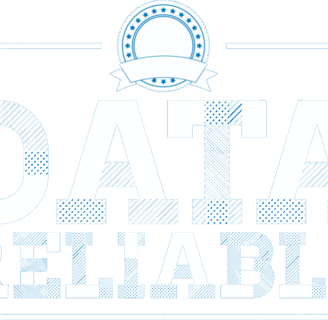